Prof. Luc Brun
ENSICAEN
3 September - Morning Session
IAPR Distinguished speaker
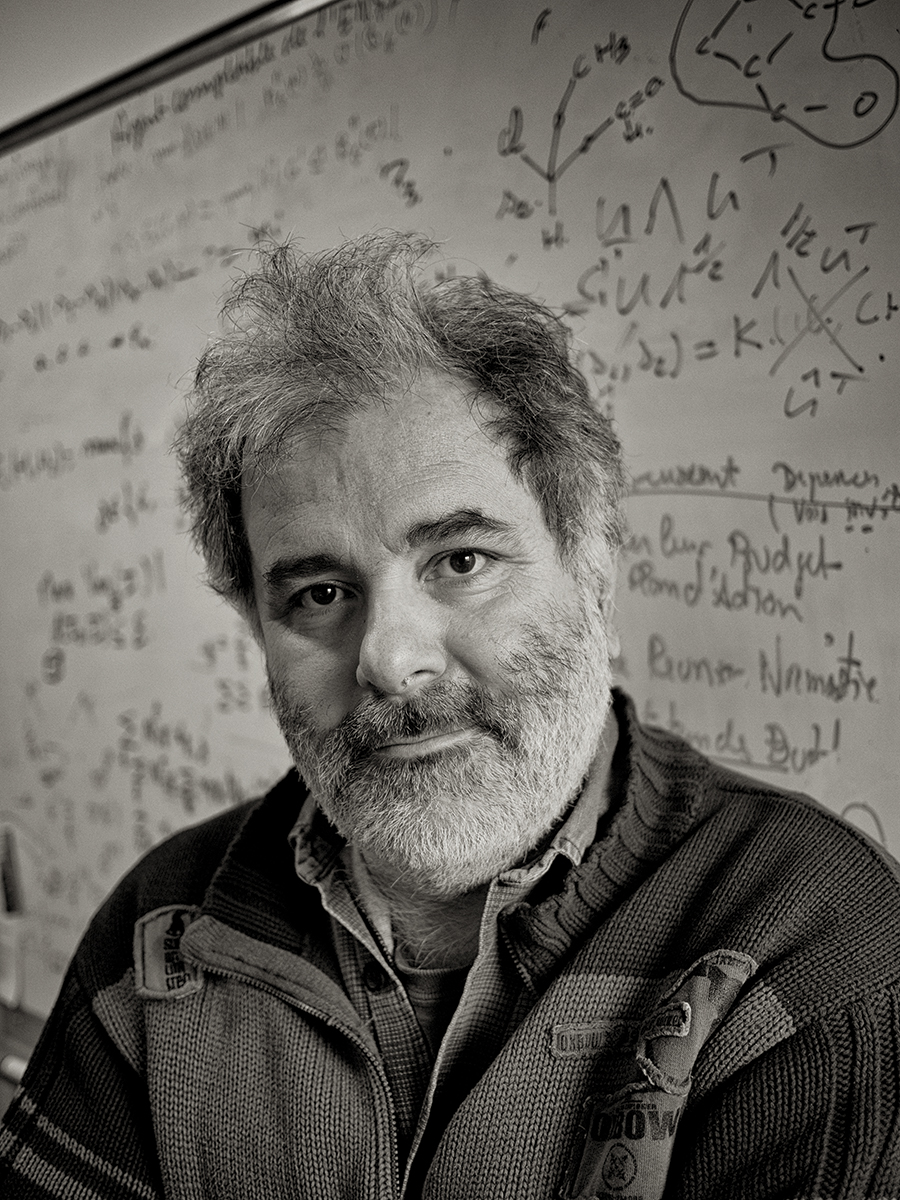
Luc Brun is a professor of computer science at the school of engineering ENSICAEN, in Caen, Normandy, France.
He received his Ph.D. in Computer Science in 1996 at the University of Bordeaux I. After few years as assistant professor at the University of Reims, he became Professor of computer science at ENSICAEN. Since this date he supervised 11 PhD students. He is currently one of the French representative at the IAPR's Governing board and is serving as chairman of the membership committee of IAPR and is the head of a research federation (NormaSTIC) grouping all research activities in computer science performed in Normandy.
Luc Brun began his carrier by designing hierarchical and non hierarchical image segmentation models on which many segmentation algorithms may be designed. The aim of this research was to provide an efficient access to photo-metrical, topological and geometrical information about an image partition using either a single or multiple scales of description. Since he became a professor, his research turned towards structural pattern recognition with several contributions concerning Graph kernels and Graph edit distance. The main application fields are chemo/bio informatics, video analysis, shape recognition.
Web page: https://brunl01.users.greyc.fr/ - E-mail: luc.brun@ensicaen.fr.
-
Graph Classification
-
Graphs are a rich data structure allowing to encode efficiently both sub parts of objects and the relationships between these sub parts. At a first glance, graphs seems to be a promising model for the classification of complex objects. Unfortunately, there is no free lunch and graphs charge for this flexibility in several ways: Firstly most of (useful) graph algorithms are either NP-complete or NP-hard. Secondly, metrics defined on graph spaces induce specific properties on these spaces which should be manipulated with care. In this talk we will review three families of graph metrics together with the properties of the associated spaces. We will also adopt a slightly different point of view and describe recent advances on Graph neural networks.
Prof. James Ferryman
University of Reading
3 September - Afternoon Session
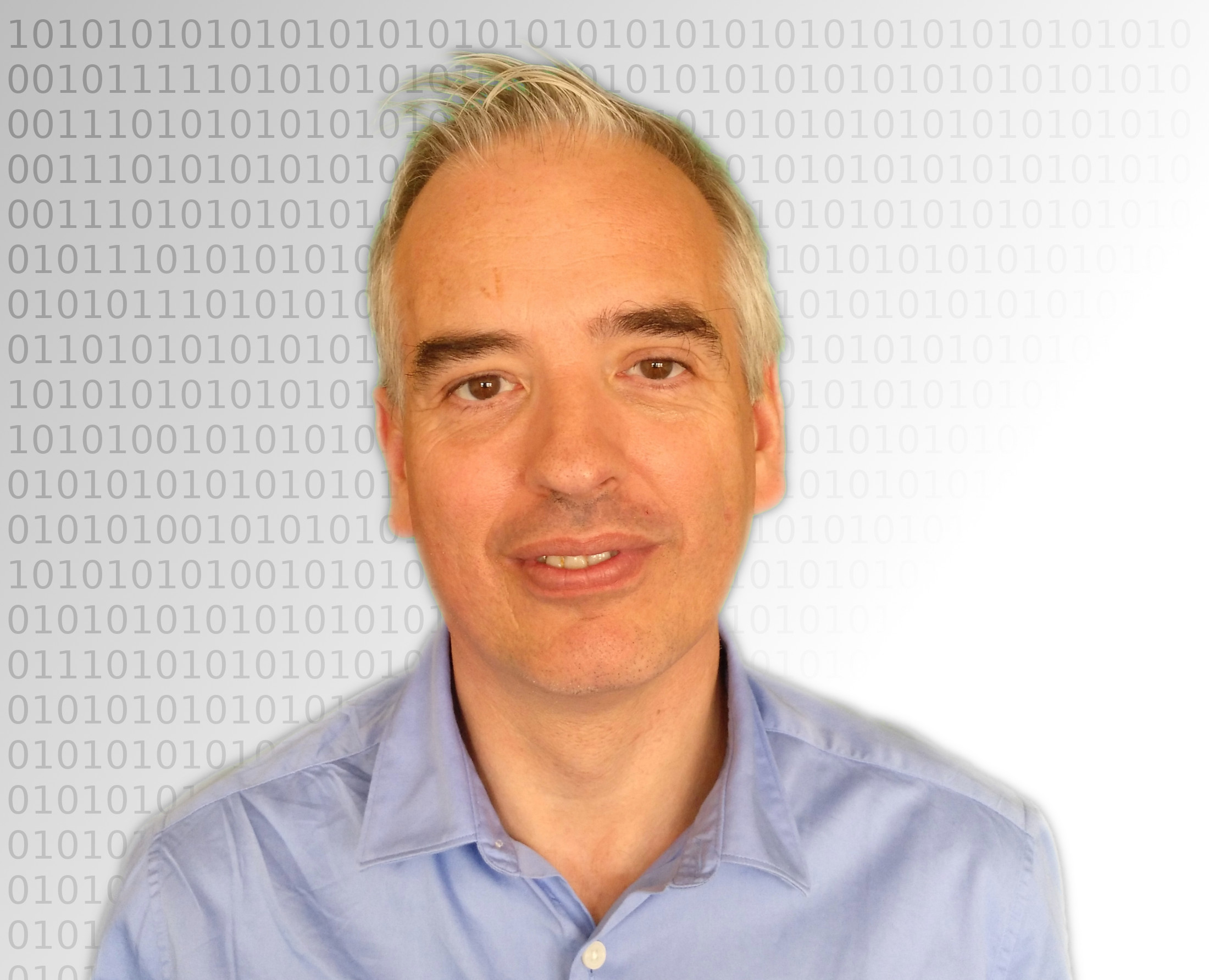
Prof. Ferryman is a computer scientist and leads the Computational Vision Group within the Department of Computer Science, School of Mathematical, Physical and Computational Sciences (SMPCS), University of Reading.
His current research interests include multimodal biometrics, automated video surveillance and benchmarking. He is the author of more than 100 scientific publications. He has participated in a wide range of UK and EU funded research programmes including, under border security, the EU EFFISEC project (FP7-217991) on efficient integrated security checkpoints, the EU IPATCH project (FP7-60756on automated surveillance and decision support system for detection and classification of piracy threats to shipping, and the EU FastPass project (FP7-312583) on development of a harmonised modular reference system for all European automated crossing points, the latter of which he led the largest technological workstream on traveller identification and monitoring. Prof. Ferryman coordinated the EU PROTECT project (H2020-700259, 2016-2019) on exploration of current and future use of biometrics in border security. Prof. Ferryman is a member of the British Computer Society and has acted as the Director of both the British Machine Vision Association and the Security Information Technology Consortium. Since 2000, he has been a Co-Chair of the IEEE International Workshop on Performance Evaluation of Tracking and Surveillance.
Web page: https://www.cs.reading.ac.uk/ - E-mail: j.m.ferryman@reading.ac.uk.
-
Biometrics and Surveillance on the Move: Vision for Border Security
-
Biometrics and video surveillance are ubiquitous. Fingerprint and face verification on smartphones has brought biometrics to the masses. Automated video surveillance is deployed in public spaces for anomalous event and behaviour detection. Biometrics, and to a lesser extent video surveillance, is also integral to border security. Automated Border Control (ABC) eGates enable automated clearance for low-risk travellers. While such systems have been transformative and have many benefits over manual controls there exist many challenges. The talk will begin by discussing these issues and determining an overall vision of border security of the future. Next, the outcomes of an EU FastPass project, which aimed to develop a harmonized approach to eGates incorporating both innovative development and application of biometrics and video surveillance, will be presented. The talk will then advance the vision by proposing contactless biometric-based, free-flowing on-the-move border control systems taking into account privacy and security issues which such innovation raises. In this context, the recently completed EC PROTECT project will be presented including the novel biometric and surveillance concepts and technical solutions that were developed, including results of two demonstrations addressing travellers in vehicles as well as on foot on-the-move. Finally, the talk will extend the vision to consider how the PROTECT work fits within the overall concept of so called “no-gate crossing solutions” and why the whole identity lifecycle of a traveller needs to be addressed.
Prof. Gian Luca Marcialis
University of Cagliari
4 September - Morning Session
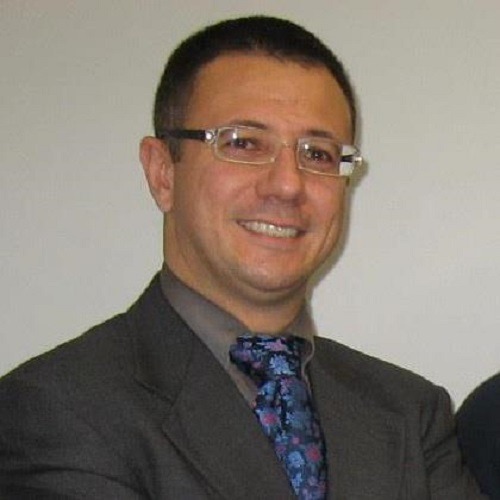
Gian Luca Marcialis is Associate Professor of Computer Engineering at the University of Cagliari, Italy. He obtained the MS degree in Electronic Engineering in 2000, and the Ph.D. degree in Computer Engineering in 2004. Since 2000 he joined the Pattern Recognition and Applications Laboratory (PRA Lab) of the Dept. of Electrical and Electronic Engineering - University of Cagliari, where he is currently director of the Lab’s Biometric Unit. His research interests are in the area of pattern recognition and its application to new and challenging tasks. During his career, Gian Luca Marcialis has published more than one hundred papers on international journals, conferences and books (H-index is 33 according to Google Scholar). His main contributions are in the field of biometrics and in particular on the multi-modal fusion of classifiers for fingerprint classification and verification, the vulnerability assessment of multiple biometric systems, face recognition, adaptive biometric systems and fingerprint liveness detection, EEG signal processing. Gian Luca Marcialis acts as a reviewer for the vast majority of the international journals on pattern recognition and applications, as well as member of the programme committee of many IEEE and IAPR international conferences. He is involved in international and national research projects as responsible and team leader, and is also co-organizer of the International Competition of Fingerprint Liveness Detection (LivDet). He teaches B.Sc. and Ph.D. courses at University of Cagliari on the topic “Biometric technologies for information security”. Gian Luca Marcialis is member of the IAPR (International Association for Pattern Recognition) and IEEE (International Association of Electrical and Electronic Engineers).
Web page: https://pralab.diee.unica.it/en/GianLucaMarcialis - E-mail: marcialis@unica.it.
-
Fingerprint Presentation Attacks Detection: from the “loss of innocence” to the “International Fingerprint Liveness Detection” competition
-
More than 15 years ago, the research community claimed that fingerprints «are very difficult to reproduce and steal». We lost our “innocence” when, between 2001 and 2002, some scholars fabricated artificial replicas of the fingers, named “fake fingers” or “gummy fingers”, that, if put on the fingerprint sensor surface, provided images impossible to distinguish from those of the live fingers, even by visual inspection of experts. This led to a plethora of countermeasures reported in journals, conference proceedings and international projects: generally speaking, pattern recognition and machine learning-based approaches, where the main problem was to find the best feature set able to describe the differences between live and fake fingerprints. Since the basic problem was the lack of data, the organization of the International Fingerprint Liveness Detection Competition, known as “LivDet”, helped our research group to acquire a strong know-how on the difficulties in fabricating fake fingers and the effectiveness of the state-of-the-art algorithms. In the 2019 edition, we tested algorithms integrating presentation attacks detectors and verification systems. It was the first time that liveness detection was handle as a part of a more complex problem, that is, the design of an “intrinsically secure” fingerprint verification system. Therefore, what is the state-of-the-art? Where are we? In this lecture, we summarize our experience on the problem and trace the path from the first fingerprint liveness detection algorithms to the most recent approaches. In particular, the “battle” between the handcrafted features and the deep learning- based paradigms which characterized the last four years of research, is pointed out. On the basis of what reported by the scientific state-of-the-art and the LivDet results over years, we show that the word “end” cannot be still said on this challenging topic.
Prof. Nicolai Petkov
University of Groningen
4 September - Afternoon Session
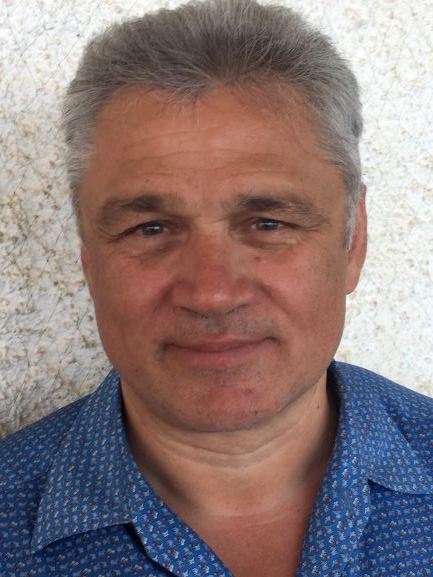
Nicolai Petkov is professor of computer science with a chair in Intelligent Systems and Parallel Computing at the University of Groningen since 1991.
He received his doctoral degree at the Dresden University of Technology in Germany. After graduation he worked at several universities and in 1991 he was appointed professor of Computer Science at the University of Groningen. He was the PhD thesis director of 32 scientists till now. He was scientific director of the Institute for Mathematics and Computer Science (now Bernoulli Institute) from 1998 to 2009.
Nicolai Petkov is associate editor of several scientific journals (e.g. J. Image and Vision Computing). He co-organized and co-chaired the 10th International Conference of Computer Analysis of Images and Patterns CAIP 2003 in Groningen, the 13th CAIP 2009 in Münster, Germany, the 16th CAIP 2015 in Valletta, Malta, the International Workshops Braincomp 2013, 2015, 2017 and 2019 on Brain-Inspired Computing in Cetraro, Italy, and the International Conference on Applications of Intelligent Systems APPIS 2018 and 2019 in Las Palmas de Gran Canaria, Spain.
Petkov's research interests are in the field of development of pattern recognition and machine learning algorithms that he applies to various types of big data: image, video, audio, text, genetic, phenotype, medical, sensor, financial, web, etc.[2] He develops methods for the generation of intelligent programs that are automatically configured using training examples of events and patterns of interest. See further www.cs.rug.nl/is.
-
Representation learning with trainable COSFIRE filters
-
Download the presentation here.
In order to be effective, traditional pattern recognition methods typically require a careful manual design of features, involving considerable domain knowledge and effort by experts. The recent popularity of deep learning is largely due to the automatic configuration of effective early and intermediate representations of the data presented. The downside of deep learning is that it requires a huge number of training examples.
Trainable COSFIRE filters are an alternative to deep networks for the extraction of effective representations of data. COSFIRE stands for Combinations of Shifted Filter Responses. Their design was inspired by the function of certain shape selective neurons in areas V4 and TEO of visual cortex. A COSFIE filter is configured by the automatic analysis of a single attern. The highly non-linear filter response is computed as a combination of the responses of simpler filters, such as Difference of (color) Gaussians or Gabor filters, taken at different positions of the concerned pattern. The identification of the parameters of the simpler filters that are needed and the positions at which their responses are taken is done automatically. An advantage of this approach is its ease of use as it requires no programming effort and little computation – the parameters of a filter are derived automatically from a single training pattern. Hence, a large number of such filters can be configured effortlessly and selected responses can be arranged in feature vectors that are fed into a traditional classifier.
This approach is illustrated by the automatic configuration of COSFIRE filters that respond to randomly selected parts of many handwritten digits. We configure automatically up to 5000 such filters and use their maximum responses to a given image of a handwritten digit to form a feature vector that is fed to a classifier. The COSFIRE approach is further illustrated by the detection and identification of traffic signs and of sounds of interest in audio signals.
The COSFIRE approach to representation learning and classification yields performance results that are comparable to the best results obtained with deep networks but at a much smaller computational effort. Notably, COSFIRE representations can be obtained using numbers of training examples that are many orders of magnitude smaller than those used by deep networks.